

Air Pollution Forecasting


This project involved forecasting Sulphur Dioxide concentrations in the atmosphere. The data was collected from certain Air Quality survey sites from the USA. The dataset contained the following details:
Date: The date when that data point was collected.
Source: Source of information- Air Quality Survey.
Site ID: Each survey site has a unique ID.
POC: Stands for “Parameter Occurrence Code”, used to distinguish different instruments that measure the same parameter at the same site (Source).
target: Max SO2 concentration of a given day at a given site.
UNITS: The unit the measurement is taken in - ppb(Parts Per Billion.)
DAILY_AQI_VALUE: Air Quality Index of the given date at a given place.
Site Name: Name of the Survey Site.
DAILY_OBS_COUNT: Total number of observations taken in a day.
PERCENT_COMPLETE: Percentage of the number of hourly observations taken in a day(24 hours).
AQS_PARAMETER_CODE: The unique code for SO2 according to AQS, which is 42401 (Source).
COUNTY: Name of the county where the survey site is located.
SITE_LATITUDE: Latitude of the survey site.
SITE_LONGITUDE: Longitude of the survey site.
Highly correlated values were dropped after observing the correlation matrix (on the right.) After doing a thorough EDA to better understand the data. I ran multiple tests like Rolling statistics to observe Stationarity, then the Dickey-Fuller Test to check the stationarity of the data.
Once it was ascertained that the data was stationary, I performed some pre-processing steps like normalizing the variables, one-hot encoding the values.
Once the data was ready, a simpleRNN model was created to forecast the time series. RMSProp optimizer was used. Since the project didn't require highly accurate prediction, I resorted to this model for faster training. The model trained for 100 epochs and achieved a minimum validation loss of 0.0000944.
Description
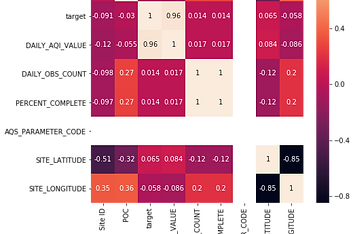
Correlation Matrix