

Nuclear Segmentation


Kaggle 2018 Science Bowl Challenge required the development of DL models that can spot nuclei in divergent images to advance medical discovery. This will catalyse the research for almost every disease, from lung cancer and heart disease to rare disorders.
The training set contained 670 coloured images, which were 128 x 128 in dimensions.
As this was a segmentation challenge, I created a Fully Convolutional Network (U-net), which would generate segmentation masks for the nuclear region. The model contained 1.9 M trainable parameters. I used Adam as the optimiser and binary cross-entropy as the loss function, because, effectively, we are predicting whether the given pixel is black or white (0 or 1).
The model Trained for 19 epochs before it stopped converging and had a validation loss of 0.0684.
Description
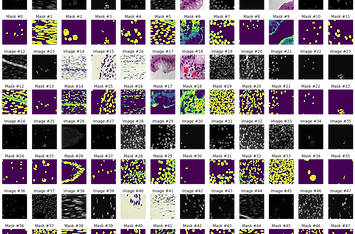
Few Samples